I like to start with exploratory data analysis to get a sense of "what the data wants to tell me" before getting into the models. If the problem related to your learning rate than NN should reach a lower error despite that it will go up again after a while. I had a model that did not train at all. Shuffling the labels independently from the samples (for instance, creating train/test splits for the labels and samples separately); Accidentally assigning the training data as the testing data; When using a train/test split, the model references the original, non-split data instead of the training partition or the testing partition. It's interesting how many of your comments are similar to comments I have made (or have seen others make) in relation to debugging estimation of parameters or predictions for complex models with MCMC sampling schemes. A place where magic is studied and practiced? How to tell which packages are held back due to phased updates. In my case the initial training set was probably too difficult for the network, so it was not making any progress. Styling contours by colour and by line thickness in QGIS. Do they first resize and then normalize the image? Thank you itdxer. You might want to simplify your architecture to include just a single LSTM layer (like I did) just until you convince yourself that the model is actually learning something. and i used keras framework to build the network, but it seems the NN can't be build up easily. Towards a Theoretical Understanding of Batch Normalization, How Does Batch Normalization Help Optimization? What should I do? learning rate) is more or less important than another (e.g. ncdu: What's going on with this second size column? Seeing as you do not generate the examples anew every time, it is reasonable to assume that you would reach overfit, given enough epochs, if it has enough trainable parameters. Without generalizing your model you will never find this issue. The nature of simulating nature: A Q&A with IBM Quantum researcher Dr. Jamie We've added a "Necessary cookies only" option to the cookie consent popup, The model of LSTM with more than one unit. By clicking Accept all cookies, you agree Stack Exchange can store cookies on your device and disclose information in accordance with our Cookie Policy. Variables are created but never used (usually because of copy-paste errors); Expressions for gradient updates are incorrect; The loss is not appropriate for the task (for example, using categorical cross-entropy loss for a regression task). Other explanations might be that this is because your network does not have enough trainable parameters to overfit, coupled with a relatively large number of training examples (and of course, generating the training and the validation examples with the same process). Does not being able to overfit a single training sample mean that the neural network architecure or implementation is wrong? To subscribe to this RSS feed, copy and paste this URL into your RSS reader. When resizing an image, what interpolation do they use? Try to set up it smaller and check your loss again. Suppose that the softmax operation was not applied to obtain $\mathbf y$ (as is normally done), and suppose instead that some other operation, called $\delta(\cdot)$, that is also monotonically increasing in the inputs, was applied instead. It took about a year, and I iterated over about 150 different models before getting to a model that did what I wanted: generate new English-language text that (sort of) makes sense. Initialization over too-large an interval can set initial weights too large, meaning that single neurons have an outsize influence over the network behavior. MathJax reference. What Is the Difference Between 'Man' And 'Son of Man' in Num 23:19? I struggled for a while with such a model, and when I tried a simpler version, I found out that one of the layers wasn't being masked properly due to a keras bug. Making statements based on opinion; back them up with references or personal experience. As you commented, this in not the case here, you generate the data only once. Do new devs get fired if they can't solve a certain bug? thank you n1k31t4 for your replies, you're right about the scaler/targetScaler issue, however it doesn't significantly change the outcome of the experiment. Usually I make these preliminary checks: look for a simple architecture which works well on your problem (for example, MobileNetV2 in the case of image classification) and apply a suitable initialization (at this level, random will usually do). In my case it's not a problem with the architecture (I'm implementing a Resnet from another paper). Data Science Stack Exchange is a question and answer site for Data science professionals, Machine Learning specialists, and those interested in learning more about the field. ), The most common programming errors pertaining to neural networks are, Unit testing is not just limited to the neural network itself. What's the difference between a power rail and a signal line? There are a number of variants on stochastic gradient descent which use momentum, adaptive learning rates, Nesterov updates and so on to improve upon vanilla SGD. For example a Naive Bayes classifier for classification (or even just classifying always the most common class), or an ARIMA model for time series forecasting. The difference between the phonemes /p/ and /b/ in Japanese, Short story taking place on a toroidal planet or moon involving flying. Then I add each regularization piece back, and verify that each of those works along the way. If your training/validation loss are about equal then your model is underfitting. +1 for "All coding is debugging". ", As an example, I wanted to learn about LSTM language models, so I decided to make a Twitter bot that writes new tweets in response to other Twitter users. Do not train a neural network to start with! I am so used to thinking about overfitting as a weakness that I never explicitly thought (until you mentioned it) that the. These bugs might even be the insidious kind for which the network will train, but get stuck at a sub-optimal solution, or the resulting network does not have the desired architecture. I just copied the code above (fixed the scaler bug) and reran it on CPU. Often the simpler forms of regression get overlooked. But these networks didn't spring fully-formed into existence; their designers built up to them from smaller units. By clicking Post Your Answer, you agree to our terms of service, privacy policy and cookie policy. How do you ensure that a red herring doesn't violate Chekhov's gun? Many of the different operations are not actually used because previous results are over-written with new variables. Also, real-world datasets are dirty: for classification, there could be a high level of label noise (samples having the wrong class label) or for multivariate time series forecast, some of the time series components may have a lot of missing data (I've seen numbers as high as 94% for some of the inputs). I think what you said must be on the right track. My model architecture is as follows (if not relevant please ignore): I pass the explanation (encoded) and question each through the same lstm to get a vector representation of the explanation/question and add these representations together to get a combined representation for the explanation and question. To subscribe to this RSS feed, copy and paste this URL into your RSS reader. I'll let you decide. Thanks for contributing an answer to Stack Overflow! 2 Usually when a model overfits, validation loss goes up and training loss goes down from the point of overfitting. Most of the entries in the NAME column of the output from lsof +D /tmp do not begin with /tmp. Here is a simple formula: $$ I have prepared the easier set, selecting cases where differences between categories were seen by my own perception as more obvious. or bAbI. In particular, you should reach the random chance loss on the test set. I couldn't obtained a good validation loss as my training loss was decreasing. For instance, you can generate a fake dataset by using the same documents (or explanations you your word) and questions, but for half of the questions, label a wrong answer as correct. Minimising the environmental effects of my dyson brain. Is this drop in training accuracy due to a statistical or programming error? (The author is also inconsistent about using single- or double-quotes but that's purely stylistic. See, There are a number of other options. I never had to get here, but if you're using BatchNorm, you would expect approximately standard normal distributions. If this works, train it on two inputs with different outputs. Some common mistakes here are. How do you ensure that a red herring doesn't violate Chekhov's gun? But why is it better? "Closing the Generalization Gap of Adaptive Gradient Methods in Training Deep Neural Networks" by Jinghui Chen, Quanquan Gu. This problem is easy to identify. Instead of training for a fixed number of epochs, you stop as soon as the validation loss rises because, after that, your model will generally only get worse . Prior to presenting data to a neural network. What am I doing wrong here in the PlotLegends specification? Sometimes, networks simply won't reduce the loss if the data isn't scaled. I think Sycorax and Alex both provide very good comprehensive answers. thanks, I will try increasing my training set size, I was actually trying to reduce the number of hidden units but to no avail, thanks for pointing out! Then make dummy models in place of each component (your "CNN" could just be a single 2x2 20-stride convolution, the LSTM with just 2 Large non-decreasing LSTM training loss. Cross Validated is a question and answer site for people interested in statistics, machine learning, data analysis, data mining, and data visualization. A recent result has found that ReLU (or similar) units tend to work better because the have steeper gradients, so updates can be applied quickly. hidden units). This is a very active area of research. Especially if you plan on shipping the model to production, it'll make things a lot easier. (One key sticking point, and part of the reason that it took so many attempts, is that it was not sufficient to simply get a low out-of-sample loss, since early low-loss models had managed to memorize the training data, so it was just reproducing germane blocks of text verbatim in reply to prompts -- it took some tweaking to make the model more spontaneous and still have low loss.). As an example, imagine you're using an LSTM to make predictions from time-series data. Alternatively, rather than generating a random target as we did above with $\mathbf y$, we could work backwards from the actual loss function to be used in training the entire neural network to determine a more realistic target. In theory then, using Docker along with the same GPU as on your training system should then produce the same results. Residual connections are a neat development that can make it easier to train neural networks. I'm not asking about overfitting or regularization. For example, suppose we are building a classifier to classify 6 and 9, and we use random rotation augmentation Why can't scikit-learn SVM solve two concentric circles? But for my case, training loss still goes down but validation loss stays at same level. "The Marginal Value of Adaptive Gradient Methods in Machine Learning" by Ashia C. Wilson, Rebecca Roelofs, Mitchell Stern, Nathan Srebro, Benjamin Recht, But on the other hand, this very recent paper proposes a new adaptive learning-rate optimizer which supposedly closes the gap between adaptive-rate methods and SGD with momentum. Loss functions are not measured on the correct scale (for example, cross-entropy loss can be expressed in terms of probability or logits) The loss is not appropriate for the task (for example, using categorical cross-entropy loss for a regression task). Can I tell police to wait and call a lawyer when served with a search warrant? By clicking Accept all cookies, you agree Stack Exchange can store cookies on your device and disclose information in accordance with our Cookie Policy. Scaling the inputs (and certain times, the targets) can dramatically improve the network's training. By clicking Post Your Answer, you agree to our terms of service, privacy policy and cookie policy. here is my code and my outputs: it is shown in Fig. Site design / logo 2023 Stack Exchange Inc; user contributions licensed under CC BY-SA. Some examples are. I used to think that this was a set-and-forget parameter, typically at 1.0, but I found that I could make an LSTM language model dramatically better by setting it to 0.25. keras lstm loss-function accuracy Share Improve this question Then training proceed with online hard negative mining, and the model is better for it as a result. Aren't my iterations needed to train NN for XOR with MSE < 0.001 too high? Then, let $\ell (\mathbf x,\mathbf y) = (f(\mathbf x) - \mathbf y)^2$ be a loss function. It become true that I was doing regression with ReLU last activation layer, which is obviously wrong. However, I am running into an issue with very large MSELoss that does not decrease in training (meaning essentially my network is not training). You have to check that your code is free of bugs before you can tune network performance! I get NaN values for train/val loss and therefore 0.0% accuracy. Does a summoned creature play immediately after being summoned by a ready action? If you want to write a full answer I shall accept it. Find centralized, trusted content and collaborate around the technologies you use most. Weight changes but performance remains the same. Note that it is not uncommon that when training a RNN, reducing model complexity (by hidden_size, number of layers or word embedding dimension) does not improve overfitting. The reason that I'm so obsessive about retaining old results is that this makes it very easy to go back and review previous experiments. Browse other questions tagged, Start here for a quick overview of the site, Detailed answers to any questions you might have, Discuss the workings and policies of this site. It could be that the preprocessing steps (the padding) are creating input sequences that cannot be separated (perhaps you are getting a lot of zeros or something of that sort). Tuning configuration choices is not really as simple as saying that one kind of configuration choice (e.g. What's the difference between a power rail and a signal line? But some recent research has found that SGD with momentum can out-perform adaptive gradient methods for neural networks. The best answers are voted up and rise to the top, Not the answer you're looking for? I understand that it might not be feasible, but very often data size is the key to success. Is it possible to rotate a window 90 degrees if it has the same length and width? To subscribe to this RSS feed, copy and paste this URL into your RSS reader. In the Machine Learning Course by Andrew Ng, he suggests running Gradient Checking in the first few iterations to make sure the backpropagation is doing the right thing. Redoing the align environment with a specific formatting. Finally, the best way to check if you have training set issues is to use another training set. Why do many companies reject expired SSL certificates as bugs in bug bounties? Use MathJax to format equations. I am writing a program that make use of the build in LSTM in the Pytorch, however the loss is always around some numbers and does not decrease significantly. If so, how close was it? Linear Algebra - Linear transformation question, ERROR: CREATE MATERIALIZED VIEW WITH DATA cannot be executed from a function. What to do if training loss decreases but validation loss does not decrease? For example you could try dropout of 0.5 and so on. If decreasing the learning rate does not help, then try using gradient clipping. Asking for help, clarification, or responding to other answers. $$. Loss is still decreasing at the end of training. rev2023.3.3.43278. number of hidden units, LSTM or GRU) the training loss decreases, but the validation loss stays quite high (I use dropout, the rate I use is 0.5), e.g.
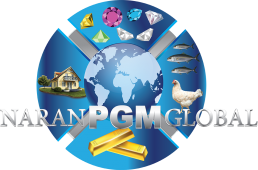